Method
In this section, we describe our unsupervised framework for monocular depth estimation. We first review the self-supervised training pipeline for monocular depth estimation, and then introduce the co-attention module and pose graph consistency loss function.
Supervision from Image Reconstruction
Following the formulation in \cite{zhou_unsupervised_2017}, the whole framework includes a DispNet and a PoseNet, the DispNet produces depth map and the PoseNet produces the relative pose between two RGB frames.
Given a sequence of consecutive frames $X_{t-1}, X_t$ and $X_{t+1}$,we estimate the depth for each frame, and the relative pose for every two adjacent frames, then we get depth map $D_{t-1}, D_t, D_{t+1}$ and translation matrix $T_{t-1\rightarrow t}, T_{t\rightarrow t+1}$.
Consider the adjacent frame pair $I_t$ and $I_{t+1}$, once the estimated depth $D_t$ and translation matrix $T_{t\rightarrow t+1}$ are available, we can project the source image $I_t$ to the next moment
$$
p(\hat{I}{t+1}) = KT{t\rightarrow t+1}D_tK^{-1}p(I_t)
$$
the function $p(.)$ denotes sampling from the homogeneous coordinates of image and $K$ denotes the camera insrinsic matrix, $\hat{I}_{t+1}$ can be reconstucted using the differentiable sampling mechanism proposed in \cite{jaderberg_spatial_2015}.
Hence the problem is formulated to the minimization of a phtometric reprojection error $L_p$
$$
L_p = \alpha \left|I_{t+1} - \hat{I}{t+1}\right|1 + (1 - \alpha)SSIM(I{t+1}, \hat{I}{t+1})
$$
$SSIM(.)$ is the structural similarity\cite{wang_image_2004} loss for evaluating the quality of image predictions, and to regularize the depth, we use a disparity image smoothness constraint as widely used in previous work\cite{mahjourian_unsupervised_2018,zhou_unsupervised_2017,garg_unsupervised_2016}
$$
L_{\mathrm{s}}=\sum_{x, y}\left|\partial_{x} D_{t}\right| e^{-\left|\partial_{x} I_{t}\right|}+\left|\partial_{y} D_{t}\right| e^{-\left|\partial_{y} I_{t}\right|}
$$
List
Here is a list:
- Xue Bai, Jue Wang, David Simons, and Guillermo Sapiro.Video SnapCut: robust video object cutout using localized classifiers. TOG, 28(3):70, 2009.
- Linchao Bao, Baoyuan Wu, and Wei Liu. CNN in MRF: Video object segmentation via inference in a CNN-based higher-order spatio-temporal MRF. In CVPR, 2018
Code
Here is some code:
def bi_search(arr:list, x:int):
l, r = 0, len(arr)
while l < r:
m = (l + r) >> 1
if arr[m] >= x: r = m
else: l = m + 1
return l
Image
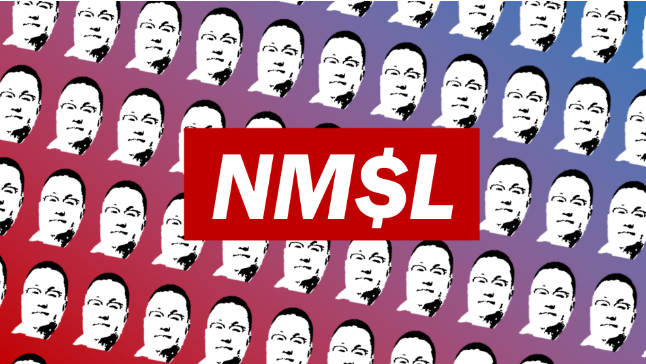
Table