我参照3DDFA更改了测试代码,结果很差,
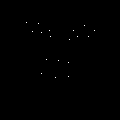
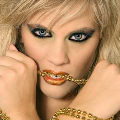
这是第4通道lmks的可视化,应该没有问题,crop方式对齐3ddfa
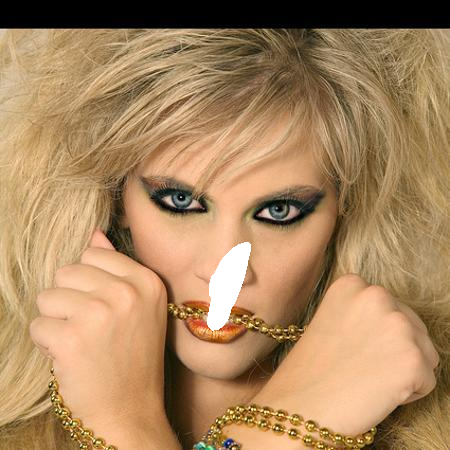
这是最终的vertex结果,白色部分是vertex,问题可能出在什么地方呢,model不是最终版本或者其他和3ddfa不同的细节之处?期待回复!!
测试main文件如下:
from utils import *
ckpt_path = 'models/2DASL_checkpoint_epoch_allParams_stage2.pth.tar'
ckpt = torch.load(ckpt_path, map_location=lambda storage, loc: storage)['res_state_dict']
state_dict = {}
for key, value in ckpt.items():
if key.startswith('module'):
state_dict[key[7:]] = value
else:
state_dict[key] = value
model = resnet50(pretrained=False, num_classes=62)
model.load_state_dict(state_dict)
transform = transforms.Compose([
ToTensorGjz(),
NormalizeGjz(mean=127.5, std=128)
])
img_path = 'test.jpg'
img = cv2.imread(img_path, cv2.IMREAD_COLOR)
lmks = get_face_lmks(img)
roi_box = parse_roi_box_from_landmark(lmks.T.copy())
img_crop = crop_img(img, roi_box)
lmks_crop = crop_lmks(roi_box, lmks)
lmks_crop = fit_lmks(lmks_crop, img_crop.shape[:2])
lmks_crop[lmks_crop>119] = 119
img_crop = cv2.resize(img_crop, dsize=(120, 120), interpolation=cv2.INTER_LINEAR)
lmks_map = get_18lmks_map(lmks_crop)
lmks_map = lmks_map[:,:,np.newaxis]
lmks_map = torch.from_numpy(lmks_map).unsqueeze(0).permute(0,3,1,2)
input = transform(img_crop).unsqueeze(0)
input = torch.cat([input, lmks_map], dim=1)
with torch.no_grad():
param = model(input)
param = param.squeeze().cpu().numpy().flatten().astype(np.float32)
dense = get_dense_from_param(param, roi_box)
print(dense.T)
show_lmks(img, dense.T)
cv2.imwrite('cache.png', img)