I use 4 gpu as follow.
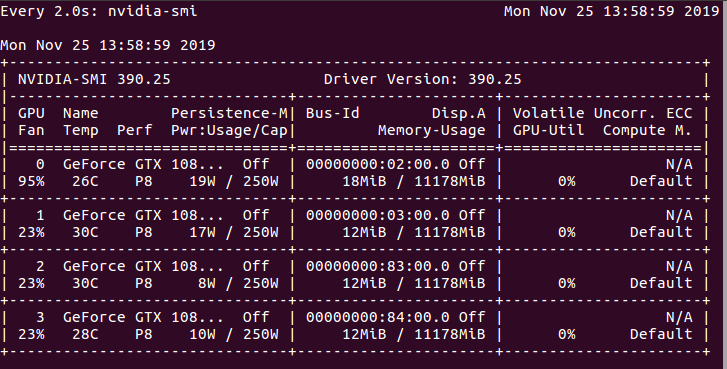
python3 train.py \
--eval --eval_dataset val \
--config ./experiments/human36m/eval/human36m_alg.yaml \
--logdir ./logs
args: Namespace(config='./experiments/human36m/eval/human36m_alg.yaml', eval=True, eval_dataset='val', local_rank=None, logdir='./logs', seed=42)
Number of available GPUs: 4
Loading pretrained weights from: ./data/pretrained/human36m/pose_resnet_4.5_pixels_human36m.pth
Reiniting final layer filters: module.final_layer.weight
Reiniting final layer biases: module.final_layer.bias
Successfully loaded pretrained weights for backbone
Successfully loaded pretrained weights for whole model
Loading data...
Experiment name: [email protected]:51:22
Traceback (most recent call last):
File "train.py", line 485, in
main(args)
File "train.py", line 478, in main
one_epoch(model, criterion, opt, config, val_dataloader, device, 0, n_iters_total=0, is_train=False, master=master, experiment_dir=experiment_dir, writer=writer)
File "train.py", line 190, in one_epoch
keypoints_3d_pred, keypoints_2d_pred, heatmaps_pred, confidences_pred = model(images_batch, proj_matricies_batch, batch)
File "/usr/local/lib/python3.6/dist-packages/torch/nn/modules/module.py", line 489, in call
result = self.forward(*input, **kwargs)
File "/home/mvn/models/triangulation.py", line 158, in forward
heatmaps, _, alg_confidences, _ = self.backbone(images)
File "/usr/local/lib/python3.6/dist-packages/torch/nn/modules/module.py", line 489, in call
result = self.forward(*input, **kwargs)
File "/home/mvn/models/pose_resnet.py", line 299, in forward
x = self.layer1(x)
File "/usr/local/lib/python3.6/dist-packages/torch/nn/modules/module.py", line 489, in call
result = self.forward(*input, **kwargs)
File "/usr/local/lib/python3.6/dist-packages/torch/nn/modules/container.py", line 92, in forward
input = module(input)
File "/usr/local/lib/python3.6/dist-packages/torch/nn/modules/module.py", line 489, in call
result = self.forward(*input, **kwargs)
File "/home/mvn/models/pose_resnet.py", line 87, in forward
out = self.bn3(out)
File "/usr/local/lib/python3.6/dist-packages/torch/nn/modules/module.py", line 489, in call
result = self.forward(*input, **kwargs)
File "/usr/local/lib/python3.6/dist-packages/torch/nn/modules/batchnorm.py", line 76, in forward
exponential_average_factor, self.eps)
File "/usr/local/lib/python3.6/dist-packages/torch/nn/functional.py", line 1623, in batch_norm
training, momentum, eps, torch.backends.cudnn.enabled
RuntimeError: CUDA out of memory. Tried to allocate 3.52 GiB (GPU 0; 10.92 GiB total capacity; 5.65 GiB already allocated; 1.96 GiB free; 2.67 GiB cached)
I use gpu that memory is 12 gigabytes, and when your model tries to allocate 3.52 gigabytes, I get an error message.
How can i solve this?